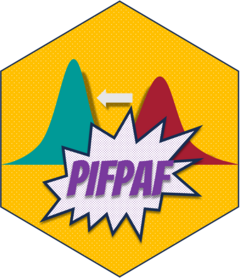
Extract confidence intervals for the potential impact fraction or the population attributable fraction
confint.pif_class.Rd
Method for obtaining confidence intervals for a pif_class
object
Arguments
- x
A
pif_class
object- parm
a specification of which parameters are to be given confidence intervals, either a vector of numbers or a vector of names. If missing, all parameters are considered.
- level
(
double
) Confidence level for the uncertainty interval. Defaults to0.95
.- method
(
string
) either'wald'
(recommended) or'percentile'
for uncertainty intervals based upon the bootstrap's percentile or the Wald-type approximation (see confidence interval section)- ...
additional methods to pass to
mean()
andstats::sd()
ifwald
orstats::quantile()
ifpercentile
type intervals
Confidence Intervals
Confidence intervals are estimated via the two methods specified by Arnab (2017) .
Wald-type confidence intervals (which are more precise) are of the form: \[ \widehat{\text{PIF}} \pm t_{1 - \alpha/2} \sqrt{\widehat{\text{Var}}_{\text{B}}\big[\widehat{\text{PIF}}\big]} \] where \(t_{1 - \alpha/2}\) stands for the percent points at level \(1 - \alpha/2\) of Student's t-distribution, and \(\widehat{\text{Var}}_{\text{B}}\big[\widehat{\text{PIF}}\big]\) represents the estimated variance (via bootstrap) of the potential impact fraction estimator.
Percentile confidence intervals (less precise) are of the form: \[ \Big[\widehat{\text{PIF}}_{\text{B},\alpha/2}, \widehat{\text{PIF}}_{\text{B},1-\alpha/2}\Big] \] where \(\widehat{\text{PIF}}_{\text{B},k}\) represents the kth sample quantile from the bootstrap simulation of the potential impact fraction estimator.
Examples
#Example 1
data(ensanut)
options(survey.lonely.psu = "adjust")
design <- survey::svydesign(data = ensanut, ids = ~1, weights = ~weight, strata = ~strata)
rr <- function(X, theta) {
exp(-2 +
theta[1] * X[, "age"] + theta[2] * X[, "systolic_blood_pressure"] / 100)
}
cft <- function(X) {
X[, "systolic_blood_pressure"] <- X[, "systolic_blood_pressure"] - 5
return(X)
}
pifsim <- pif(design,
theta = log(c(1.05, 1.38)), rr, cft,
additional_theta_arguments = c(0.01, 0.03), n_bootstrap_samples = 10,
)
confint(pifsim)
#> counterfactual relative_risk potential_impact_fraction
#> 1 Counterfactual_1 Relative_Risk_1 -0.01341996
#> 2 Counterfactual_1 Relative_Risk_1 0.03826853
#> average_relative_risk average_counterfactual type
#> 1 -1697.331 -1645.505 Lower 2.5%
#> 2 2686.984 2606.830 Upper 97.5%